AI-Powered Concept Maps: A Researcher’s Tool for In-Depth Exploration
Sep 24, 2024
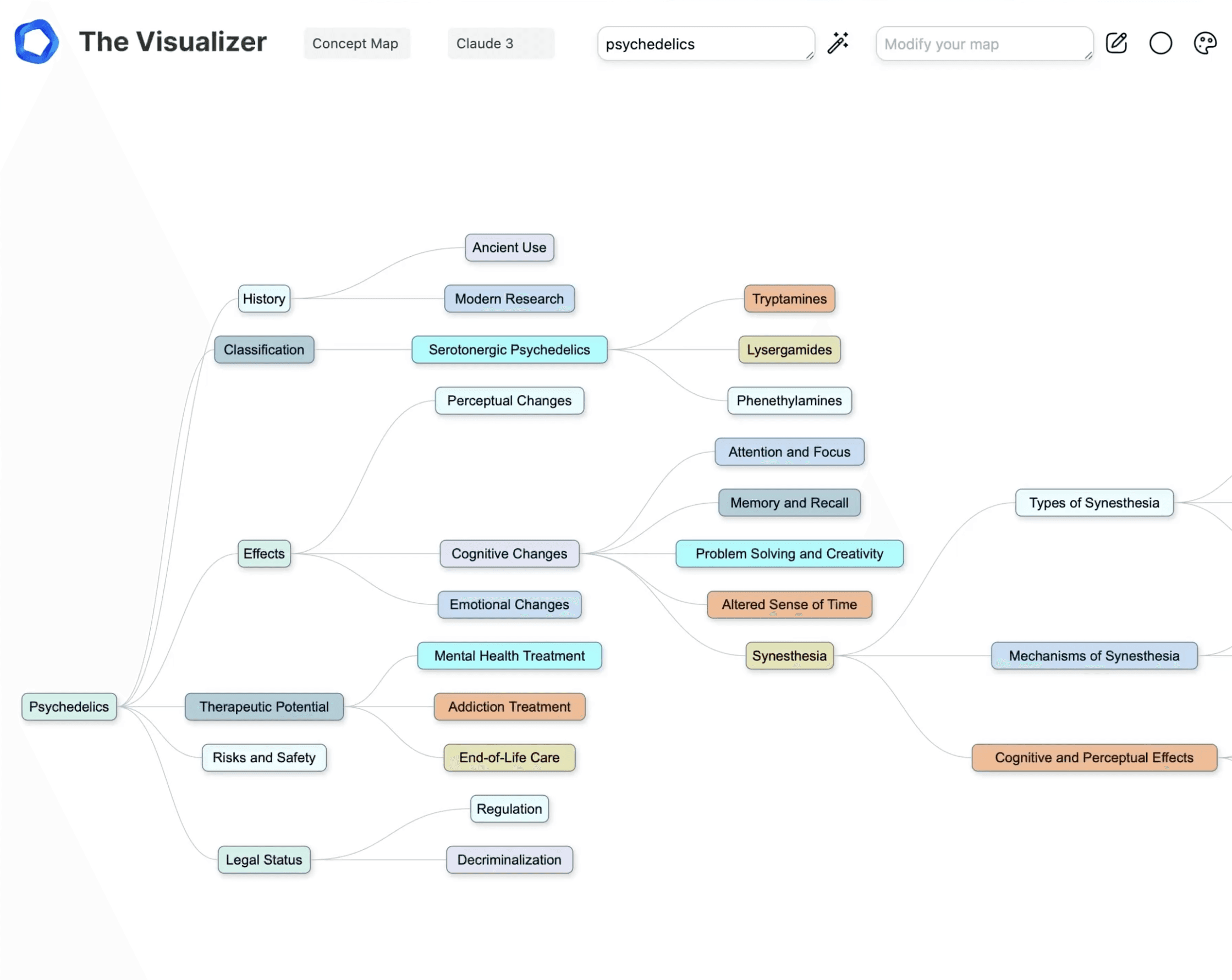
For researchers, uncovering connections between ideas and navigating complex topics often leads down intricate paths of information. AI-powered concept maps provide an innovative approach to exploring these paths, offering the ability to expand nodes and connections endlessly, while integrating AI for clarification along the way.
How AI-Powered Concept Maps Support Research
Researchers frequently engage in multidisciplinary inquiries where patterns and relationships between concepts need to be clearly visualized and comprehended. Traditional tools like mind maps offer only a static overview of ideas. However, AI-powered concept maps bring a dynamic, interactive approach that is particularly beneficial in research contexts. Here’s why:
• Infinite Node Expansion: Unlike traditional tools, AI-powered concept maps allow researchers to keep expanding ideas and their relationships without predefined limits. This makes it possible to explore topics thoroughly, especially in fields where new information continuously builds on existing knowledge, such as biology, cognitive sciences, and artificial intelligence research.
• AI-Powered Clarifications: One of the most significant benefits is the integration of AI, which allows researchers to ask for clarifications directly within the concept map. The AI can provide explanations, background context, or suggest further areas of exploration based on the researcher’s current trajectory.
Enhancing Cognitive Processes for Researchers
Concept maps have long been a tool for learning and understanding complex information. Research has shown that concept mapping improves critical thinking and knowledge retention, making it especially useful in academic and professional research . AI-powered maps extend this by facilitating multimodal learning, combining visual exploration with active inquiry through AI assistance.
Several studies support the effectiveness of concept maps in research and complex problem solving:
• Novak’s Theory on Concept Maps (2010): Concept maps are valuable for organizing knowledge in ways that enable deeper understanding and insight. Novak emphasizes their role in creating structured knowledge frameworks that help individuals to identify and link concepts .
• Chen et al. (2020) on AI in Concept Mapping: AI integration in concept mapping enhances learning by providing dynamic feedback and personalized suggestions, which helps users identify connections they may not have previously considered .
• Studies on Visualization and Cognitive Load (Paas et al., 2003): Visual tools like concept maps help reduce cognitive load when analyzing complex information. By spreading cognitive resources across visual and textual representations, researchers can improve processing efficiency .
Practical Benefits for Researchers
Researchers, particularly in interdisciplinary fields, can benefit from AI-powered concept maps in several practical ways:
• Mapping Complex Domains: In fields like molecular biology, psychology, or AI development, where knowledge is continually evolving, concept maps provide a flexible tool for expanding and updating research models without the constraints of linear diagrams.
• Identifying Novel Connections: By visually linking concepts and allowing for infinite expansion, researchers can uncover novel relationships between ideas or datasets that may have been overlooked using traditional methods. AI’s ability to assist in recognizing patterns further accelerates this process.
• Clarifying Uncertain Areas: Often in research, unclear or ambiguous topics arise. The AI function helps by offering real-time clarification, reducing the need to search external resources constantly. This keeps the researcher in the flow of idea generation and exploration.
Conclusion
For researchers, AI-powered concept maps represent an advanced tool for exploration, expanding connections ad infinitum and offering crucial AI-driven clarifications. By blending visual thinking with machine intelligence, this tool is particularly suited for those engaged in deep, multidisciplinary research, where insights and connections evolve continuously.
References:
1. Novak, J. D., & Cañas, A. J. (2010). The theory underlying concept maps and how to construct them. Technical Report IHMC CmapTools.
2. Hay, D. B., Kinchin, I. M., & Lygo-Baker, S. (2008). Making learning visible: The role of concept mapping in higher education. Studies in Higher Education, 33(3), 295-311.
3. Chen, C. C., Wang, H. F., & Chen, K. T. (2020). The impact of AI on enhancing concept mapping for learning complex subjects. Journal of Educational Technology, 15(2), 345-362.
4. Paas, F., Renkl, A., & Sweller, J. (2003). Cognitive load theory and instructional design: Recent developments. Educational Psychologist, 38(1), 1-4.